Exploring the Future of Data Science: Trends and Innovations
The Rise of Automation in Data Science
In recent years, automation has become a cornerstone of innovation in data science. As datasets grow larger and more complex, the need for automated tools to streamline processes and enhance productivity becomes increasingly evident. Automation tools can handle repetitive tasks such as data cleaning, preprocessing, and even some aspects of model training, allowing data scientists to focus on more strategic and creative aspects of their work.
This trend is driven by the development of advanced machine learning algorithms and AI frameworks, which enable faster and more accurate data analysis. As these technologies continue to evolve, we can expect automation to play an even larger role in shaping the future of data science.

The Advent of Explainable AI
As AI models become more sophisticated, the demand for transparency and understanding of these models has given rise to the field of Explainable AI (XAI). Explainable AI aims to make AI systems more transparent by providing insights into their decision-making processes. This is particularly important in sectors like healthcare, finance, and law, where understanding the reasoning behind a decision is crucial.
Techniques such as LIME (Local Interpretable Model-Agnostic Explanations) and SHAP (SHapley Additive exPlanations) are being developed to help data scientists and stakeholders understand how complex models make predictions. The focus on XAI is expected to grow as organizations seek to build trust with users and comply with regulatory requirements.

Integration of Data Science with IoT
The Internet of Things (IoT) is generating massive amounts of data from connected devices worldwide. Integrating data science with IoT allows organizations to gain deeper insights into device performance, user behaviors, and operational efficiencies. This integration supports real-time analytics, predictive maintenance, and improved decision-making processes.
Data scientists are increasingly leveraging IoT-derived data to create smarter systems and applications. As IoT devices continue to proliferate, the synergy between data science and IoT will become even more integral to technological advancement.
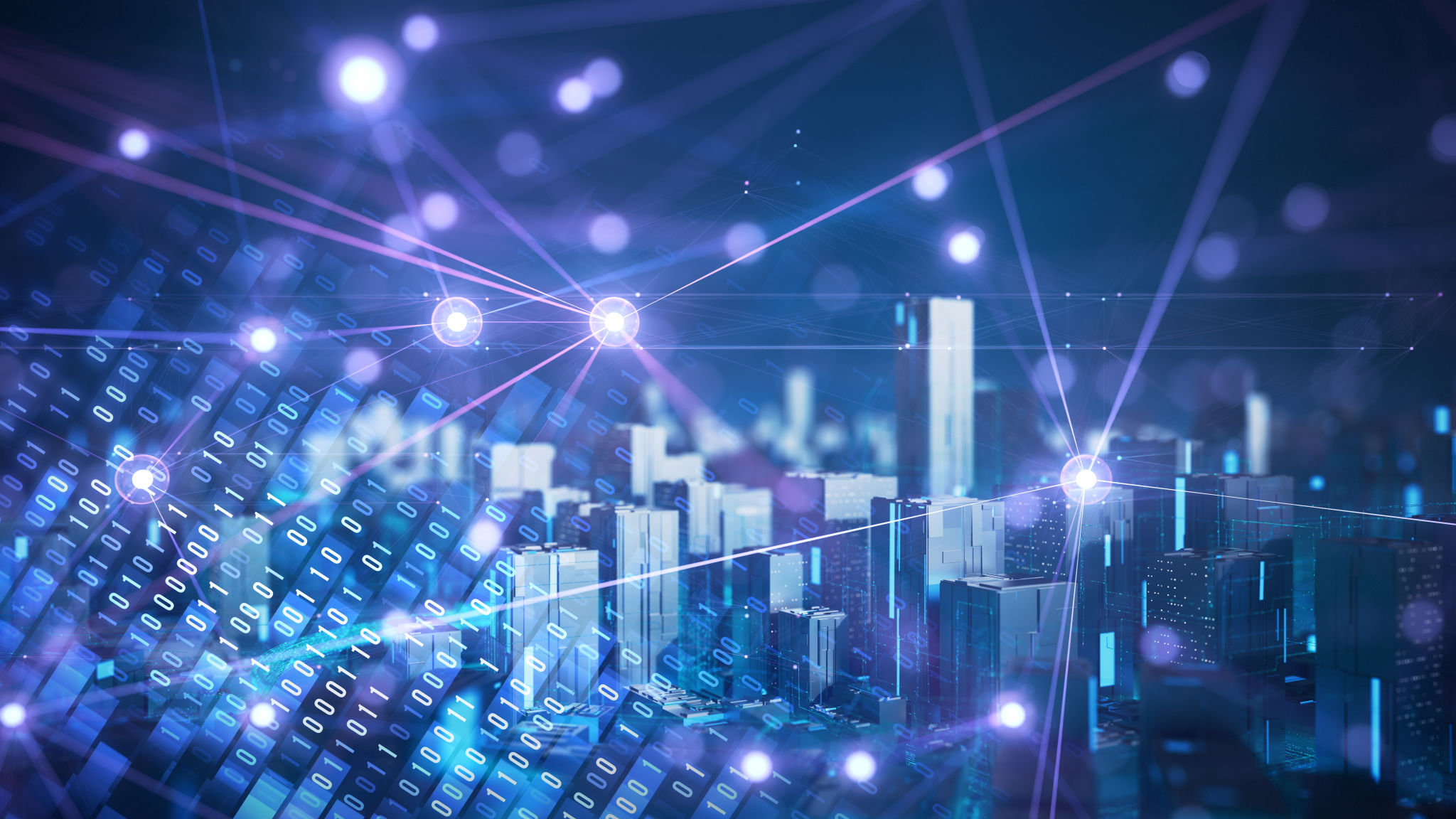
Focus on Data Privacy and Ethics
With the growing reliance on data-driven decision-making, concerns about data privacy and ethical use have come to the forefront. Data scientists are now tasked with ensuring that data collection, storage, and analysis adhere to privacy regulations such as GDPR and CCPA. Ethical considerations are crucial, especially when dealing with sensitive information that could impact individuals' lives.
Organizations are implementing strict protocols and employing privacy-preserving techniques such as differential privacy and federated learning to safeguard data. The focus on ethics in data science is set to intensify as public awareness and regulatory pressures increase.
The Expansion of Edge Computing
Edge computing is transforming the way data is processed by bringing computation closer to the source of data generation. This approach reduces latency, increases speed, and enhances the efficiency of real-time processing. For data science applications, edge computing can support faster insights and enable decision-making at the edge of networks.
As more devices become interconnected, especially in smart cities and autonomous vehicles, the demand for edge computing solutions will grow. The future will likely see more sophisticated models being deployed at the edge, unlocking new possibilities for innovation.

Enhanced Collaboration through Open Source Tools
The open-source movement has been pivotal in democratizing access to powerful data science tools. Communities around open-source platforms like TensorFlow, PyTorch, and Apache Spark have fostered collaboration and innovation at an unprecedented scale. These tools allow data scientists from diverse backgrounds to contribute to and benefit from shared knowledge.
The future of data science will likely see further collaboration as more organizations contribute to open-source projects. This collective effort will drive the development of new tools and techniques that push the boundaries of what is possible in data science.
In conclusion, the future of data science is bright with trends like automation, explainable AI, IoT integration, ethical considerations, edge computing, and open-source collaboration paving the way for new innovations. As these trends continue to evolve, they will redefine how we interact with data and shape our world.