Importance of Social Determinants of Health features in ML modeling
Introduction
When it comes to modeling the determinants of health, Machine Learning (ML) has proven to be a powerful tool. ML algorithms can analyze vast amounts of data and identify patterns that help us understand the complex factors that influence our well-being. In recent years, the importance of incorporating social determinants of health (SDOH) features in ML modeling has gained significant attention.
The Role of Social Determinants of Health
Social determinants of health are the conditions in which people are born, grow, live, work, and age. These factors include socioeconomic status, education, employment, social support networks, access to healthcare, and the physical environment. They play a crucial role in shaping our health outcomes and can have a profound impact on individuals and communities.
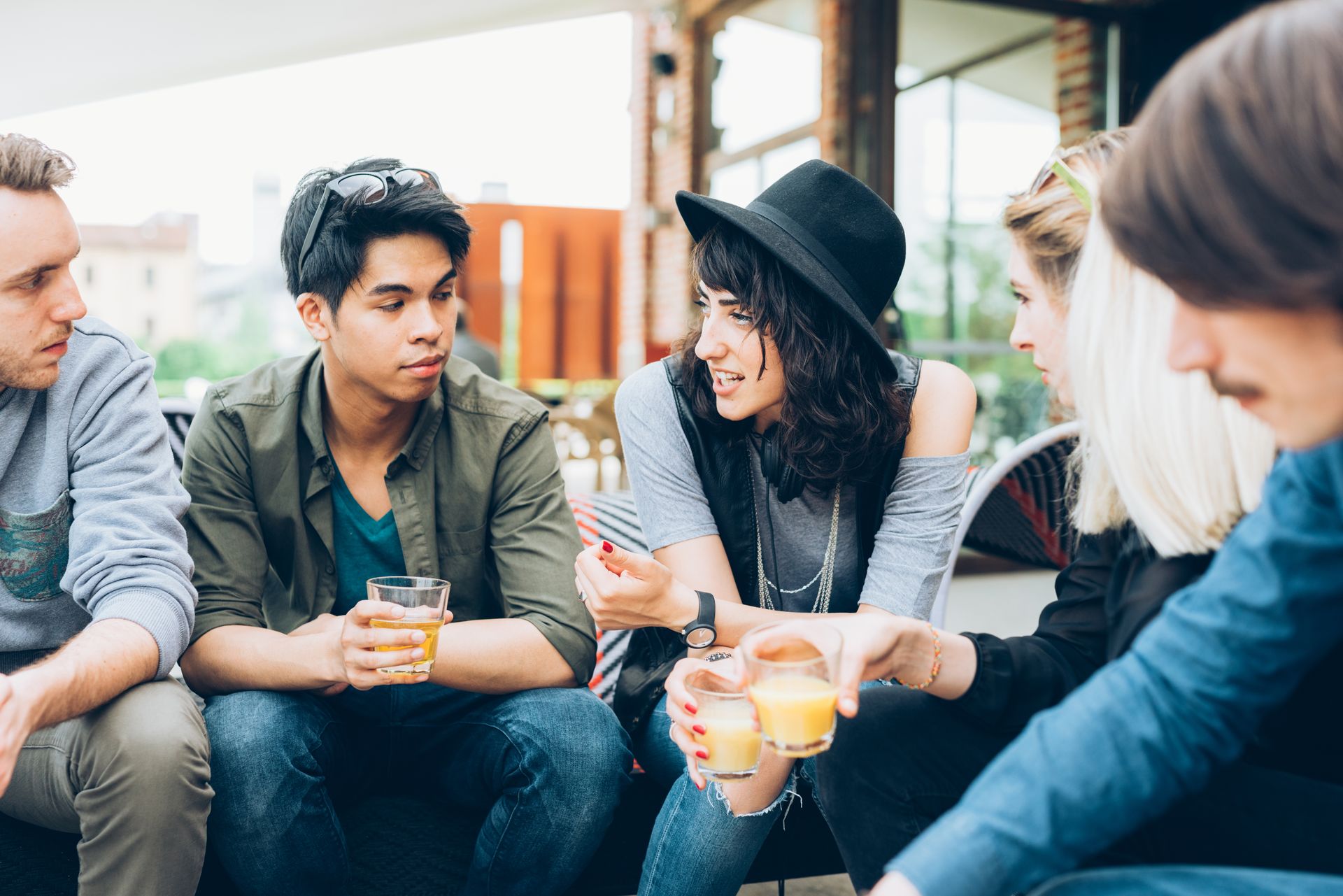
Enhancing Predictive Accuracy
By incorporating SDOH features into ML models, we can improve their predictive accuracy and gain deeper insights into the complex relationships between social factors and health outcomes. Traditional health risk models often focus solely on clinical and genetic data, neglecting the significant influence of social factors. ML models that consider SDOH features provide a more comprehensive view of an individual's health status and can predict outcomes more accurately.
Uncovering Hidden Patterns
ML algorithms excel at identifying hidden patterns and relationships in data. By including SDOH features, we can uncover correlations between social factors and health outcomes that might otherwise go unnoticed. For example, ML models might reveal that individuals with limited access to healthy food options are more likely to develop chronic diseases, or that neighborhoods with higher crime rates experience higher rates of mental health issues.
Improving Health Equity
One of the key benefits of incorporating SDOH features in ML modeling is the potential to improve health equity. By understanding how social factors impact health outcomes, we can develop targeted interventions and policies to address disparities. ML models can help identify at-risk populations and inform resource allocation, enabling healthcare providers and policymakers to prioritize interventions that promote health equity.
Data Collection Challenges
While incorporating SDOH features in ML modeling is promising, it comes with its own set of challenges. One major hurdle is the availability and quality of data. SDOH data is often fragmented, collected by various agencies and organizations, and may not be readily accessible. Additionally, ensuring the privacy and security of sensitive social data poses significant ethical considerations.
Collaboration and Data Integration
To overcome these challenges, collaboration and data integration are crucial. Healthcare providers, social service organizations, and researchers need to work together to collect and share data effectively. By integrating various sources of SDOH data, ML models can provide a more comprehensive understanding of the social determinants of health and their impact on different populations.
Policy Implications
The incorporation of SDOH features in ML modeling also has important policy implications. Policymakers can utilize ML models to evaluate the effectiveness of existing interventions and design evidence-based policies that address the root causes of health disparities. ML can provide valuable insights into the potential outcomes of different policy scenarios, enabling policymakers to make informed decisions that promote health equity.
Conclusion
As ML continues to revolutionize healthcare, incorporating social determinants of health features in modeling is essential for a more comprehensive understanding of health outcomes. By leveraging the power of ML algorithms and integrating SDOH data, we can improve predictive accuracy, uncover hidden patterns, and work towards a more equitable healthcare system that addresses the social factors influencing our well-being.
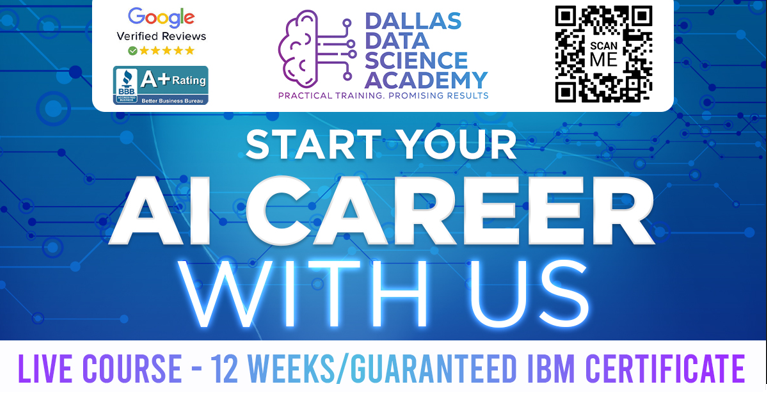
Dallas Data Science Academy stands out for its distinctive approach of LIVE mentoring, offering individualized attention and immersive hands-on training through real-life projects guided by practicing Data Scientists based in the USA. Our excellence reflects in the numerous 5-star Google reviews from a vast array of contented students. Secure your spot for our free sessions by visiting DallasDataScienceAcademy.com/Classes. Join us to shape your AI journey!