Integrating Generative AI in Data Science Projects
Understanding Generative AI
Generative AI is a subset of artificial intelligence focused on creating new content, such as text, images, and even music. Unlike traditional AI models that predict outcomes based on input data, generative AI algorithms are designed to produce novel outputs. This capability can be particularly powerful in data science projects where the need for innovation and experimentation is paramount.
The core of generative AI lies in models like Generative Adversarial Networks (GANs) and transformers. These models learn patterns from existing data and generate new data with similar characteristics, making them ideal for tasks such as data augmentation, simulation, and creative problem-solving.
The Role of Generative AI in Data Science
Incorporating generative AI into data science projects can significantly enhance the scope and efficiency of these initiatives. By automating the generation of synthetic data, data scientists can address challenges like data scarcity and imbalance, which are common in real-world datasets. This ability to create high-quality synthetic datasets allows for more robust model training and validation.
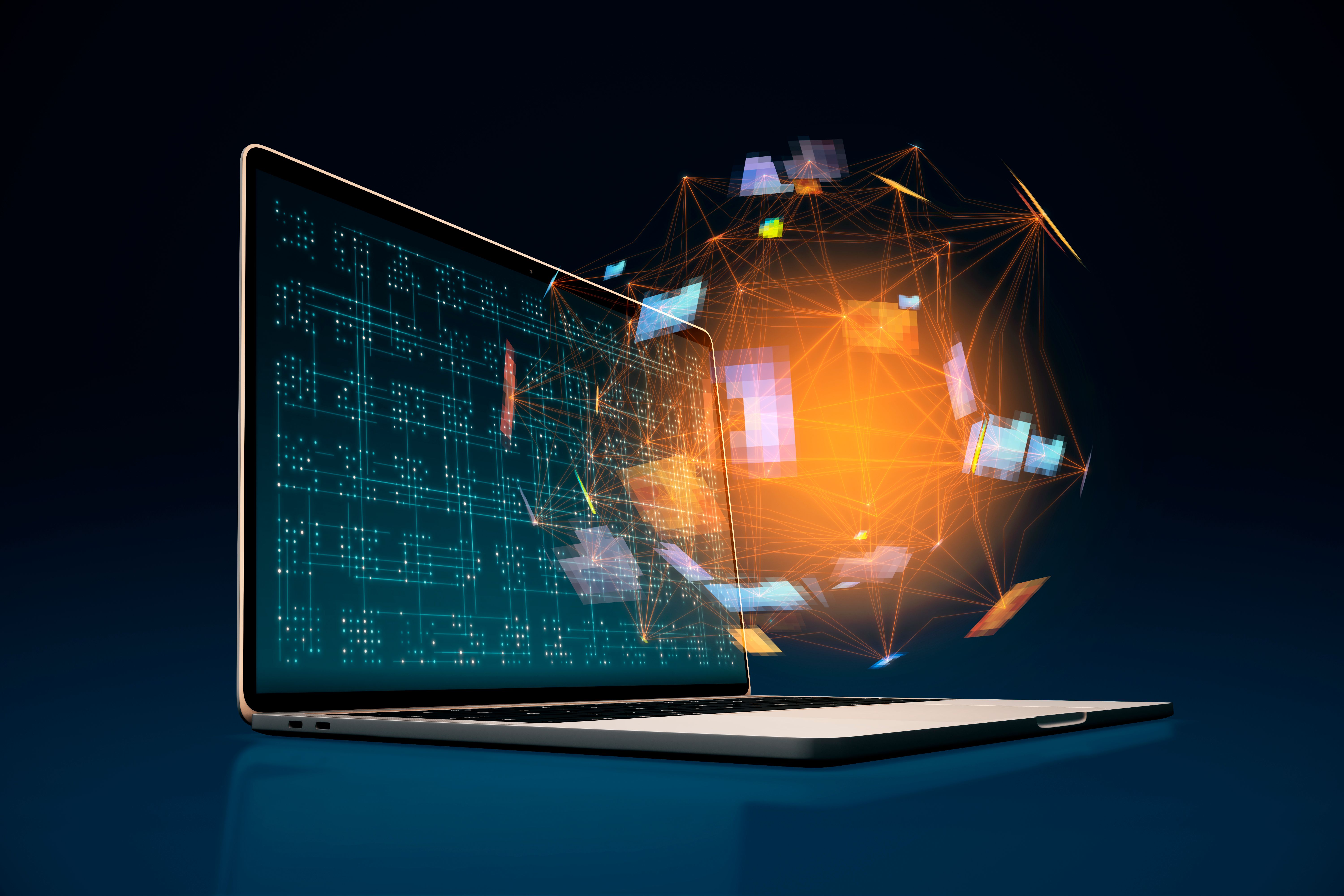
Data Augmentation
One of the primary uses of generative AI in data science is data augmentation. By generating additional training examples, generative models help improve the accuracy and generalization of machine learning algorithms. This process is particularly beneficial for tasks such as image recognition, where diverse data is crucial for model success.
Enhancing Predictive Analytics
Generative AI can also enhance predictive analytics by simulating various scenarios and outcomes. This capability is especially useful in industries like finance and healthcare, where understanding potential future trends can lead to better decision-making. By integrating generative models into predictive analytics pipelines, organizations can gain deeper insights and improve forecasting accuracy.
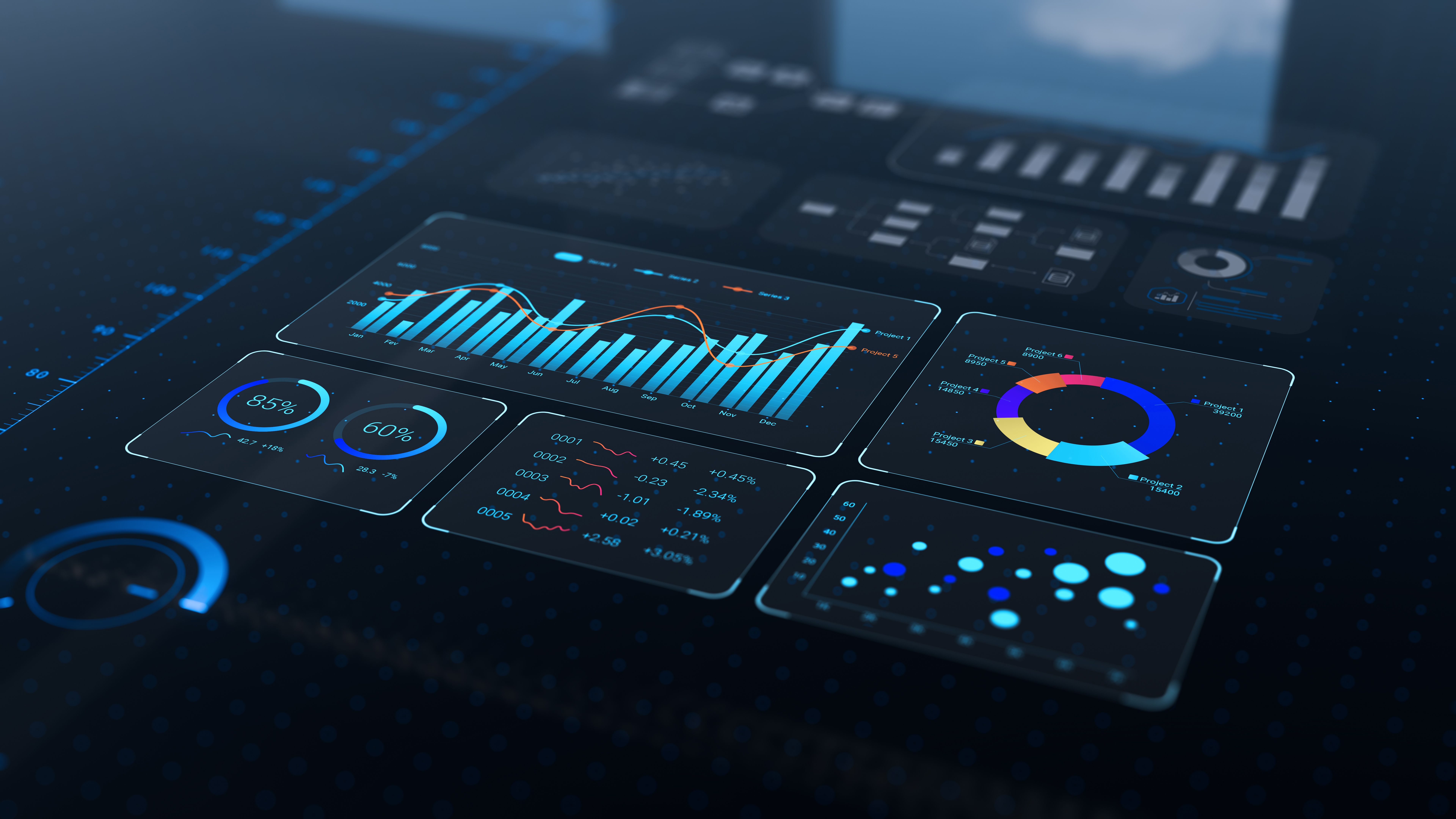
Automated Feature Engineering
Feature engineering is a critical step in the data science workflow, often requiring significant time and domain expertise. Generative AI can automate this process by identifying and creating new features that improve model performance. This not only saves valuable time but also uncovers patterns that may not be immediately apparent to human analysts.
Implementing Generative AI in Your Projects
To successfully integrate generative AI into your data science projects, start by identifying areas where it can add the most value. Consider factors such as data availability, project goals, and existing infrastructure. Once these areas are identified, select appropriate generative models that align with your objectives.
Implementing these models requires a combination of technical expertise and creativity. Collaborate with cross-functional teams to ensure the integration aligns with business objectives and technical capabilities. Moreover, continuously evaluate the impact of generative AI on project outcomes to refine and optimize its use.
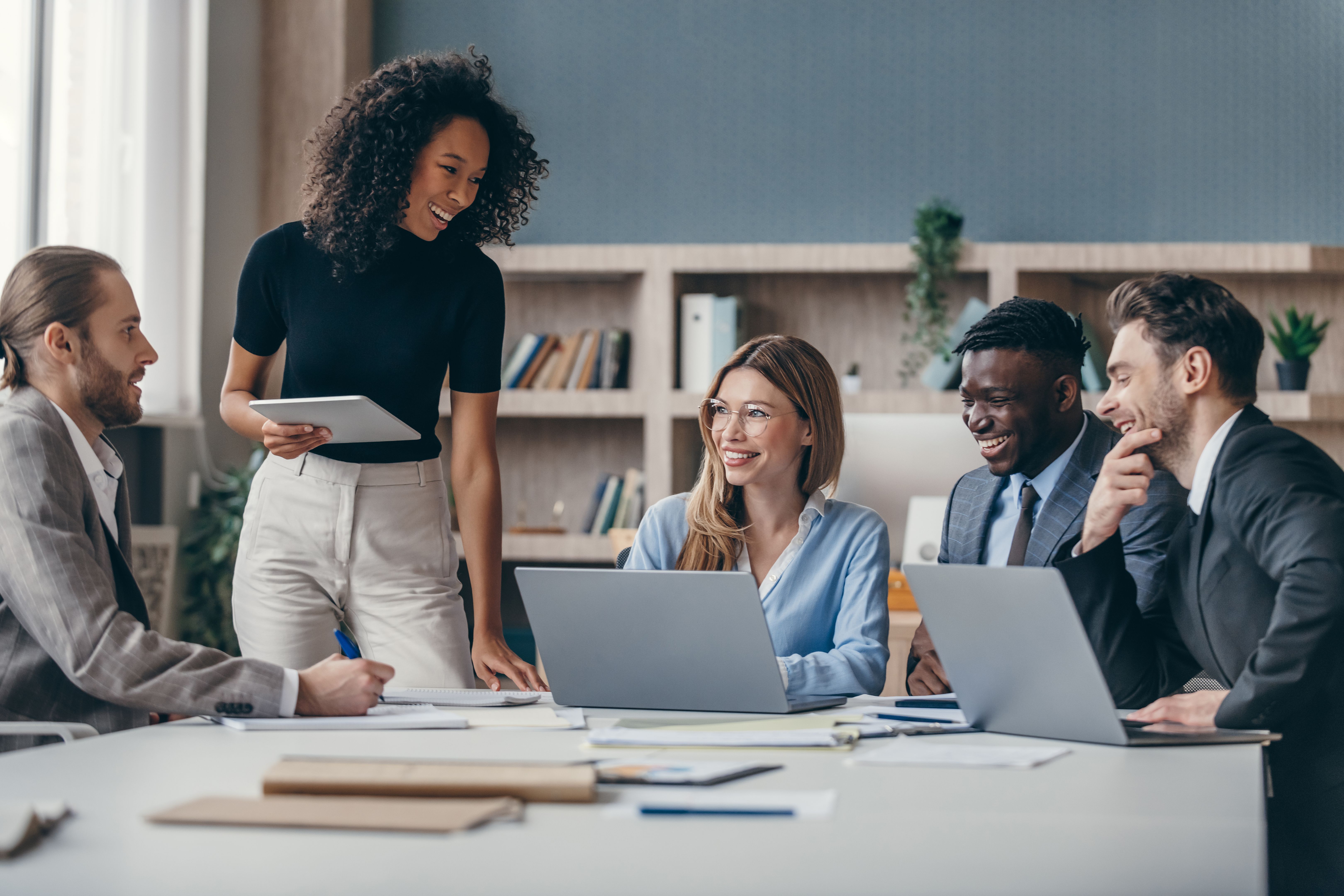
Challenges and Considerations
While generative AI offers numerous benefits, it also presents challenges. Ensuring the quality and relevance of generated data can be difficult, especially when working with complex datasets. Ethical considerations must also be addressed, particularly when generating data that mimics real-world entities or behaviors.
Despite these challenges, the integration of generative AI in data science projects continues to evolve, offering exciting opportunities for innovation and discovery.