Statistics in Data Science
Statistics is a fundamental component of data science, providing the necessary tools and techniques to analyze and interpret data. It plays a crucial role in extracting meaningful insights and making informed decisions. In this blog post, we will explore the significance of statistics in data science and its various applications.
The Importance of Statistics in Data Science
Statistics is the backbone of data science, enabling us to make sense of complex datasets. By applying statistical methods, data scientists can identify patterns, trends, and relationships within the data. This knowledge is essential for developing predictive models, understanding customer behavior, and making data-driven decisions.
Descriptive Statistics
Descriptive statistics is the branch of statistics that focuses on summarizing and describing data. It involves calculating measures such as mean, median, and standard deviation to provide a concise overview of the data. These statistics help data scientists gain a better understanding of the central tendencies and variability within the dataset.
Inferential Statistics
Inferential statistics involves drawing conclusions and making predictions about a population based on a sample of data. This branch of statistics allows data scientists to make generalizations and test hypotheses using techniques like hypothesis testing and confidence intervals. It helps in making predictions and understanding the uncertainty associated with those predictions.
Applications of Statistics in Data Science
Data Exploration and Visualization
Statistics plays a crucial role in data exploration and visualization. By analyzing the distribution of variables, data scientists can identify outliers, missing values, and potential data quality issues. They can also create visualizations such as histograms, scatter plots, and box plots to gain insights into the data and communicate findings effectively.
Machine Learning
Statistics forms the foundation of machine learning algorithms. Techniques such as regression, classification, and clustering rely on statistical principles to make predictions and identify patterns in the data. By understanding statistical concepts, data scientists can select appropriate models, evaluate their performance, and fine-tune their parameters to achieve accurate results.
A/B Testing
A/B testing is a statistical technique commonly used in data science to compare the performance of two or more variants. By randomly assigning users to different groups and measuring their responses, data scientists can determine the effectiveness of different strategies or interventions. This helps businesses optimize their products, marketing campaigns, and user experiences.
Conclusion
Statistics plays a vital role in data science, providing the necessary tools and techniques to analyze, interpret, and make predictions from data. It enables data scientists to uncover valuable insights, develop accurate models, and make informed decisions. By leveraging statistical methods, data scientists can unlock the full potential of data and drive meaningful outcomes.
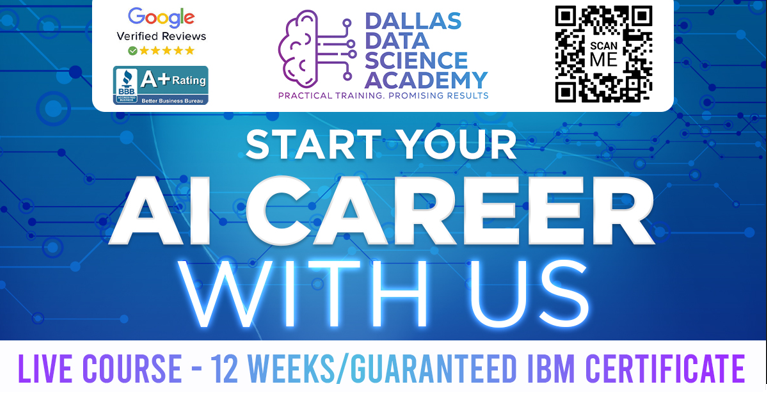
Dallas Data Science Academy stands out for its distinctive approach of LIVE mentoring, offering individualized attention and immersive hands-on training through real-life projects guided by practicing Data Scientists based in the USA. Our excellence reflects in the numerous 5-star Google reviews from a vast array of contented students. Secure your spot for our free sessions by visiting DallasDataScienceAcademy.com/Classes. Join us to shape your AI journey!