Understanding the Differences: AI Governance vs. Machine Learning Governance
Understanding the Differences: AI Governance vs. Machine Learning Governance
With the explosion of artificial intelligence (AI) applications, establishing strong governance frameworks is crucial. However, the terms "AI governance" and "Machine Learning (ML) governance" are often misunderstood or used synonymously. Let's unpack these concepts to see how they differ and how they fit together for responsible AI management.
AI Governance: Setting the Big Picture Rules
Think of AI governance as the overarching framework governing how an organization adopts, develops, uses, and monitors all its AI systems. Key focuses include:
Ethical AI: How AI aligns with principles like fairness, non-discrimination, transparency, and accountability.
Compliance: Adherence to data privacy laws (GDPR, etc.), and emerging AI-specific regulations.
Risk Assessment: Identifying potential harms, including cybersecurity threats, biases, or unintended consequences.
Strategic Alignment: Ensuring AI projects support business goals while mitigating broader social or economic risks.
ML Governance: The Technical Nitty-Gritty
ML governance is a vital subset of the broader AI governance framework. It focuses specifically on the processes, policies, and technical controls surrounding the development and management of machine learning models.
Key concerns include:
Data Quality: Ensuring training and testing data is representative, unbiased, and adheres to privacy regulations.
Model Development: Using sound methodologies, avoiding overfitting, documenting model choices, and tracking model versions.
Interpretability: Prioritizing explainable models (especially in high-stakes scenarios), identifying decision-making logic.
Deployment and Monitoring: Tracking model performance, detecting drift or degradation, and having re-training or decommissioning procedures.
Why Does the Distinction Matter?
Understanding the difference between these two helps us see why both are essential and have a unique focus:
AI Governance Specialists need a broad perspective, grappling with ethical questions, regulatory landscapes, and the potential impact of AI beyond specific models.
ML Governance Specialists often have a more technical background, ensuring individual models are accurate, reliable, and well-documented throughout their lifecycle.
Collaboration is Key
In practice, AI and ML governance teams work closely together. AI governance sets the broad principles, while ML governance specialists ensure that individual models are developed and deployed in line with those broader goals.
The Future of Responsible AI
Evolving Regulations: Expect more specific AI regulations globally, making AI governance professionals a necessity for businesses of all sizes.
Explainability as Paramount: As AI becomes woven more deeply into decision-making processes, the ability to explain AI outcomes will be non-negotiable, putting ML governance at the forefront.
Cross-Industry Need: Responsible AI adoption isn't just for tech companies. Every industry utilizing AI needs tailored AI and ML governance protocols.
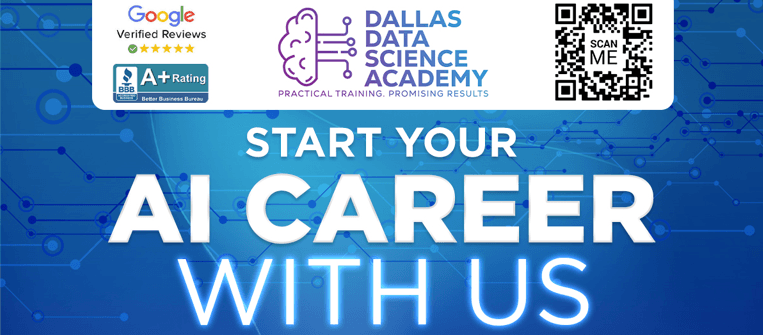
Dallas Data Science Academy stands out for its distinctive approach of LIVE mentoring, offering individualized attention and immersive hands-on training through real-life projects guided by practicing Data Scientists based in the USA. Our excellence reflects in the numerous 5-star Google reviews from a vast array of contented students. Secure your spot for our free sessions by visiting DallasDataScienceAcademy.com/Classes. Join us to shape your AI journey!